AMD's AI Accelerator Business: Puts and Takes
Bears, bulls, and timing. It’s all about the long-term view.
AMD’s 60% CAGR guidance for the AI accelerator TAM is something of a double-edged sword. On one hand, it reinforces confidence in the long-term expansion of the AI market. On the other, it implicitly suggests that AMD’s Instinct growth will track similarly.
AMD recently decided to stop breaking out Instinct revenue, suggesting they don’t want to be boxed into a 60% growth target for 2024 or 2025. This has triggered a wave of skepticism from analysts and investors wondering if Instinct is underperforming relative to management’s initial expectations.
Is 60% too ambitious? If so, what should the number be?
This leads to a more fundamental question: should we be bullish or bearish on Instinct?
Instinct Bulls
A typical but naive Instinct bull case goes something like this: Nvidia held a de facto AI GPU monopoly, but with AMD’s Instinct offerings now available, hyperscalers will welcome an alternative to reduce their reliance on Nvidia and counter its pricing power.
Instinct Bears
The bears have many valid counterarguments.
1) Instinct systems are not comparable substitutes to Nvidia
The argument is that an Nvidia AI datacenter is non-fungible; AMD-based AI systems aren’t a competitive substitute for Nvidia.
Sure, MI300X silicon is performant and MI300X has more HBM capacity than H100 (important for massive LLMs).
But, an AI system is the sum of its parts–software, hardware, and networking–and AMD’s software and networking put AMD’s overall system performance at a disadvantage to Nvidia, especially for training.
2) Nvidia’s inertia
Customers are already using Nvidia. Why change? An object in motion stays in motion.
The installed base is massive, and the software tooling is already there and easy to use. What about AMD’s Instinct systems will overcome this inertia and convince AI engineers to switch?
3) Nvidia’s speed
Nvidia isn’t standing still.
Look at Nvidia’s 2026 roadmap: next-generation memory, compute, networking innovations with Rubin GPU with HBM4, Vera CPU, and NVLink 6. And Nvidia keeps improving their software too, from Nvidia’s blog
As a result of continued software improvements, the Hopper architecture delivered up to 27% more inference performance compared to the prior round [of MLPerf].
The goalposts are moving.
How can AMD compete across the entire system, from compute to memory to networking to software, with far fewer R&D resources than Nvidia? Can they punch above their weight?
4) Nvidia’s previous-gen instances may be cost-competitive with AMD
As Blackwell ramps up, Hopper prices will continue to fall. Although training demands bleeding edge performance from Blackwell, some inference workloads are “fast enough” on Hopper.
Hyperscalers already augment their capacity with GPU clouds like CoreWeave, Lambda, and Applied Digital; what if Hopper prices fall enough to be cost-competitive with Instinct systems? Won’t some customers stick with previous-gen Hopper instead of switching to AMD and ROCm? If it ain’t broke, don’t fix it.
5) AI ASICs
Customers want to reduce Nvidia’s pricing power, sure. But who says customers will reach for another GPU? What if they instead reach for an AI ASIC?
This seems to be the case with Nvidia’s largest companies who are developing custom AI accelerators (AI ASICs or XPUs). Examples include Google's TPU, Meta's MTIA, Amazon's Trainium and Inferentia, and Microsoft's Maia. Broadcom and Marvell have stated they have multiyear roadmaps with these customers.
Is it the case that the few companies buying most of GPUs will continue to buy Nvidia and custom ASICs, not Instinct?
Note that internal custom ASICs aren’t the only alternative – don’t forget AI accelerators from startups. For example, just a few days ago, Perplexity launched a model on Cerebras-powered infrastructure:
Starting today, all Perplexity Pro users will be able to try out the latest version of Sonar, Perplexity's in-house model that is optimized for answer quality and user experience. Powered by Cerebras inference infrastructure, Sonar runs at a blazing fast speed of 1200 tokens per second — enabling nearly instant answer generation.
Granted, startups like Cerebras and Groq have a long way to go too, as Perplexity’s CEO made clear:
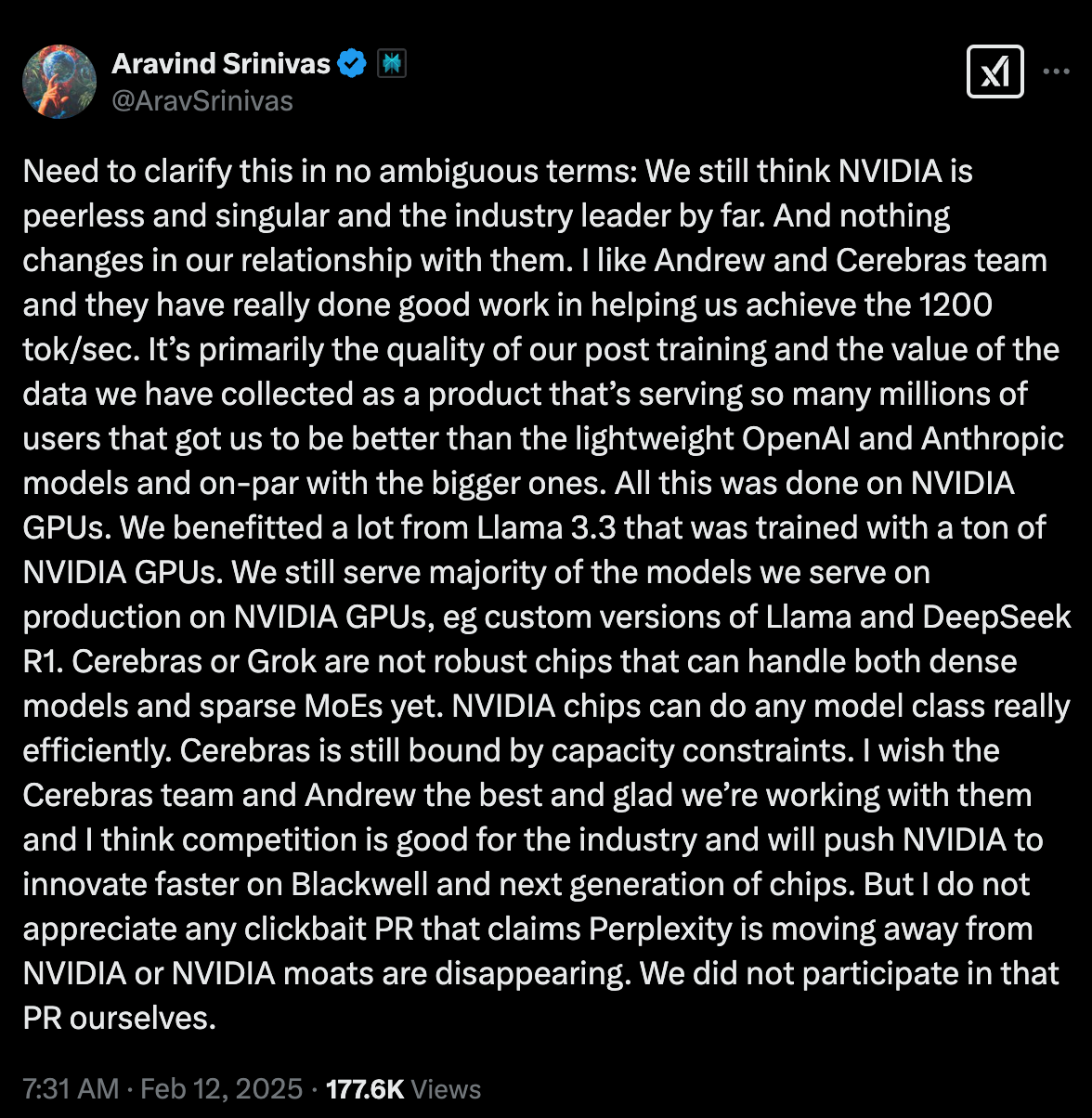
Instinct has to battle next-gen Nvidia, previous-gen Nvidia, hyperscaler internal XPUs, and startup AI accelerators. 🥴
Is there a legitimate future for AMD’s Instinct business?
Or is this simply an Nvidia market?
Long-Term View - AMD Puts
But wait! There are legitimate reasons to have AMD optimism in the long run, which we will walk through below.
But let’s first acknowledge Instinct bulls’ timing is way too optimistic.
As a reference point: How long did it take AMD to chip away at Intel’s x86 share?
It took 20+ quarters of grinding to get to 20% market share. Five years.
If server CPU market share took five years to approach the leader, what makes anyone think AI accelerators will be any different, or even faster?
But it’s investors who have the timing wrong, not AMD. Building up an AI accelerator business from scratch is a marathon. And that’s exactly what Lisa has been saying. From her June 2024 interview with Ben Thompson,
LS: I would say it a different way, we are at the beginning of what AI is all about. One of the things that I find curious is when people think about technology in short spurts. Technology is not a short spurt kind of sport, this is like a 10-year arc we’re on, we’re through maybe the first 18 months. From that standpoint, I think we’re very clear on where we need to go and what the roadmap needs to look like.
Lisa is playing the long game.
The challenge, then, is to build a compelling case for why AMD’s methodical approach will ultimately pay off. What arguments can we make for AMD making strides in the long run?
1) ASICs are not perfect substitutes for GPUs
Broadcom’s CEO Hock Tan dropped a bombshell on their Dec ‘24 earnings call:
As you know, we currently have three hyper-scale customers who have developed their own multi-generational AI XPU road map to be deployed at varying rates over the next three years. In 2027, we believe each of them plans to deploy one million XPU clusters across a single fabric. We expect this to represent an AI revenue serviceable addressable market, or SAM, for XPUs and network in the range of $60 billion to $90 billion in fiscal 2027 alone.
Investors took this at face value without thinking through the nuances.
Let’s slow down and think this through from first principles.
Q: Who is buying AI accelerators?
A: Mostly Microsoft, AWS, Meta, Google.
All of these hyperscalers use the GPUs for internal workloads, and Microsoft, AWS, and Google also rent out these GPUs to third party developers.
Given Hock’s proclamation, it’s natural to wonder: if XPUs are good enough for the hyperscaler’s internal LLM workloads, won’t they turn around and rent their XPUs out to others? Heck, Anthropic is already moving their training and inference workloads to AWS’ custom ASICs — isn’t that case in point?
Well, it’s unclear if Anthropic is making this switch for performance and cost reasons or rather because their patron saint told them to. Yes, we’ll fund your eye-watering pretraining scaling race. Here’s $4B; we expect you’ll spend it on Trainium/Inferentia credits.
Q: What motivates AI accelerator purchases?
A: LLM training and inference, specifically pretraining scaling.
Pretraining scaling dominates purchasing decisions. We have evidence of inference scaling laws too (i.e. test-time scaling with reasoning models), but inference demand is only beginning to ramp.
Q: Which AI accelerators are being purchased?
A: Mostly GPUs, save for Google Gemini on TPUs.
AWS also has a significant Trainium/Inferentia installed base, but is only starting to serve meaningful traffic on them; per the AWS keynote in December:
First, let’s talk about Claude 3 P5 Haiku that Peter just mentioned. It’s one of the newest and fastest models. Despite its small size, it packs a punch, sometimes matching the performance of our largest model, Opus, while costing 15 times less.
As Peter mentioned, we worked together to build this latency-optimized mode that lets customers run Haiku even faster on Trainium 2. This means, as of today, you can run Haiku 60% faster. No changes needed on your side—you just flip a switch on the API, and your requests get routed to the new Trainium 2 servers. Easy.
Note this isn’t the Claude production app serving consumers on AWS, but third-party developers. Also I find it a bit interesting they are using Trainium2 for inference here; why not Inferentia? It kind of ruins the chip naming convention 😅 Maybe that signals Trainium 2 systems are very performant.
Okay, speaking of fun stuff, I have something to announce. You see, so far, we’ve been focusing on inference, but they don’t call it Trainium for nothing. I’m excited to announce that the next generation of Claude will train on Project Rainier, a new Amazon cluster with hundreds of thousands of Trainium 2 chips.
Hundreds of thousands of Trainium 2 is quite meaningful, and one can squint and imagine a future where that increases 4-5x.
But,
Q: What must be true if three companies deploy 1MM+ XPUs in 2027?
Hock implies three of the four will happen in 2027:
Google: 1MM TPUs for Gemini and third-party devs
AWS: 1MM Trainium/Inferentia for Claude and third-party devs
Microsoft: 1MM Maia for OpenAI and third-party devs
Meta: 1MM MTIA for Llama training and internal workloads
Is that possible? Only if
Scaling laws continue
LLM training shifts from GPUs to XPUs
Companies are willing to accept the risk tradeoffs of XPUs
Scaling Laws Must Continue
Are we sure we’ll actually have enough data to keep scaling pretraining? At NeurIps, Ilya implies pre-training is dead:
Hardware scale alone doesn’t drive progress—without fresh training data, those million XPUs won’t translate into better models.
If Hock is betting on inference-driven demand by 2027, that’s an optimistic take—but are inference algorithms mature enough to justify locking them into fixed-function silicon?
Training must shift to GPUs
Will three companies by 2027 shift all training to XPUs?
Google’s Gemini was trained on TPUs, so that’s believable.
Anthropic’s Claude was trained on GPUs, but will seemingly move to Trainium 2. We don’t actually know if Trainium 2 systems are competitive for large LLM training, but Anthropic speaks very highly of them, so we’ll assume they are.
This still implies Microsoft (OpenAI) or Meta would move to XPU-only training by 2027. I haven’t seen Meta mention using MTIA for LLM training yet, but rather as designed for deep learning recommendation models.
MTIA is a long-term venture to provide the most efficient architecture for Meta’s unique workloads… specifically our deep learning recommendation models that are improving a variety of experiences across our products…designed to efficiently serve the ranking and recommendation models that provide high-quality recommendations to users.
If current MTIA systems are tailored for deep learning recommendation models, they might not run LLMs faster than GPUs.
Side note: I searched Meta Careers for “LLM” and “GenAI” and saw mention of Nvidia for LLM training.
I also saw hints of LLMs on MTIAs, and mention of AMD:
These jobs are a reminder that XPUs have to compete end-to-end. It’s not simply about the chip architecture — it’s still about the scale-up and scale-out networking, the software, the developer ecosystem, and so on.
And it’s unclear how many of these custom ASIC systems are competitive with GPUs (yet).
Companies are willing to accept the risk tradeoffs of XPUs
Yes, custom ASICs can have significantly better power, performance, and cost efficiency for specific workloads, but at the cost of flexibility.
Are we sure the software innovations have settled down enough to invest billions to design and manufacturing 1MM+ fixed-function chips? If they’ll be deployed in 2027, they’re being actively designed right now.
What software innovations are just around the corner that might not work well on a custom ASIC? GPUs, given their flexibility, can support such architectural innovations and optimizations.
GPUs have a large surface area for absorbing software innovations. Custom ASICs, not so much.
Let’s get technical.
Let’s say the ASIC is very tailored to transformer LLM workloads. Is hardwiring special circuitry for the self-attention mechanism risky? Attention is a fundamental performance unlock for generative AI, so that’s here to stay right?
Likewise, is it risky to build custom memory hierarchies and access patterns for KV caches? They are essential for improving the latency and throughput of transformers. Won’t we always need those?
Well, it turns out the computational and memory requirements of self-attention grow quadratically with the length of the input sequence. Want to feed in 1M+ tokens to a multimodal transformer model, like feeding in a recording of the Super Bowl so you can ask questions about the game and the commercials? Given quadratic scaling, that’s going to require terabytes of memory.
Consequently, researchers are exploring alternative architectures that offer linear scaling, such as state-space sequence models (SSMs) like Mamba. In theory, linear scaling means 1M input tokens would only require MBs of memory.
If innovations like state-space sequence models take-off, would they run on transformer ASICs? Probably (it’s all matrix multiplication, right?) But the circuitry for self-attention would be dead silicon, the memory for KV cache might not be used, and the overall dataflow and memory access patterns might actually be less efficient than just implementing SSMs on GPUs.
Now, not all ASICs are going to be quite that custom, some may be a bit more flexible, like Google’s TPU. But, the more flexible, the more like a GPU it is and the less performance and power gains.
And don’t get me wrong; there’s a place for LLMs and accelerators. Google is clearly successful with TPUs. It’s also worth noting there’s a spectrum of flexibility; Google’s TPUs are still fairly flexible compared to, say, a transformer-architecture-specific chip from Etched. (See this for great insight into TPUs).
Given the flexibility trade-offs, XPUs may not be the drop-in replacement on the roadmap as folks believe Broadcom.
If the architectural innovation isn’t well shaped for the particular custom ASIC, then the ASIC is not a substitute for GPUs.
AMD’s GPUs can be a perfect substitute for Nvidia GPUs if AMD can catch up on system performance, developer experience, and software ecosystem.
In my opinion, those Broadcom 2027 SAM dollars are at play, and AMD is in the game.
2) Cost optimization is not yet a priority.
AMD wants to compete on TCO, but TCO hasn’t been a priority — yet.
So far, GPU demand has been fueled by the race to scale pretraining, yet pretraining is at a plateau right now.
In the meantime, inference demand is showing signs of life – ChatGPT usage is growing, reasoning models are driving increased inference compute needs, and DeepSeek demonstrates optimizations for inference workloads.
With the scaling of inference demand comes a rising need for performance and cost efficiency. Inference is about balancing tokens per dollar, throughput, latency, and utilization. Accordingly, we’re starting to see some of the largest inference workloads being cost and performance-optimized right now — and the big dogs like Meta, Microsoft, and OpenAI are choosing Instinct.
As Lisa Su explained in the recent earnings call,
Meta exclusively used MI300X to serve their Llama 405B frontier model on meta.ai and added instinct GPUs to its OCP-compliant Grand Teton platform, designed for deep learning recommendation models and large-scale AI inferencing workloads.
Microsoft is using MI300X to power multiple GPT 4-based Copilot services and launched flagship instances that scale up to thousands of GPUs for AI training and inference and HPC workloads.”
At the moment, OpenAI is driving the majority of inference workloads. If they are exploring cost optimization with AMD, that’s a very good sign.
And Meta and Microsoft are two of the top three largest GPU installed base and are exploring inference cost optimization with AMD — another great sign.
Again, I think Broadcom’s 2027 SAM is at play. Take a look at these value props:
Nvidia: flexibility + premium performance + time-to-market
AMD: flexibility + competitive performance + TCO
XPUs: TCO + premium/competitive performance (TBD)
By 2027, might AMD’s software improvements enable customers to get market as easily and quickly as Nvidia? And could system performance, training including, compete with Nvidia?
3) AMD can make enough software progress in the long run
AMD has acknowledged the need to improve ROCm since late 2023. Yet throughout most of 2024, progress remained opaque. It’s lip-service unless you show and tell!
The lack of public awareness regarding tangible ROCm improvements meant the only available signal was frustrated developer posts online. This created a perception problem: whether or not AMD made improvements, the narrative was shaped by upset developers experiencing ROCm pain points.
But the tide is turning! AMD is starting to own the narrative, mainly thanks to Anush Elangovan and his public evangelism.
Software Culture, Communication Improving
Anush, the founder and former CEO of nod.ai—acquired by AMD in late 2023—has emerged as the voice of AMD’s AI software team.
He has taken on a public evangelism role, working to persuade the AI engineering community that AMD is committed to improving ROCm.
There’s no better spokesperson to talk to software engineers about ROCm improving than a software engineer working on improving ROCm.
I’ll paste some tweets below — they are mostly replies to others, so read the quoted (bottom) tweet first and then see Anush’s responses to get a sense of his servant-minded, dev-centric evangelism:
That’s textbook customer service too.
By the way, why does it matter that Anush is out canvassing X? Because he’s talking to his audience where they already are!
Hobbyists and ROCm
Take note of the following tweet. AMD is asking which gaming graphics cards developers would like ROCm support for:
It seems small, but this is really important. Home AI experimentation is a boon for Instinct. Many developers and hobbyists want to use ROCm on gaming GPUs as affordable hardware for tinkering with AI. These users want to train models, run AI applications, and experiment without breaking the bank. These folks are vital to a robust open-source ROCm ecosystem. And today’s hobbyists (undergrads, PhD researchers, curious developers) are tomorrow’s decision-makers.
This is a page from Nvidia’s playbook. Nvidia’s CUDA supports the full range of Nvidia GPU hardware, from integrated graphics to top-tier accelerators, and has had backward compatibility for quite some time.
AMD clearly recognizes that they need to support more GPUs like Nvidia, but note it’s a significant investment to support older architectures that weren’t built with backward compatibility in mind. That said, AMD is at least open to making this happen.
Hyperscalers
Companies with the biggest inference workloads (and, therefore, the strongest incentive to cut costs) also tend to have the in-house expertise to optimize for alternative software stacks. Meta, Microsoft, and OpenAI are prime examples of this trend. These are the very customers who will push the ROCm ecosystem the most, driving improvements and potentially even contributing back to its development.
Software Innovations
Software innovations like ZML, which allow developers to write their neural networks once and run them anywhere (Nvidia, AMD, Google TPU, AWS, Trainium) make developing high-performance inference applications for AMD hardware easier than ever. ZML's effectiveness is already evident in its role helping AI startup poolside port to Tranium/Inferentia:
Long Term Mindset
AMD’s developer ecosystem progress is as evident as ever.
But let's not forget: this is a marathon. It’s a five-year journey. ROCm isn’t of the woods, but progress is progress.
4) AMD can compete at the system level in the long run
Nvidia's NVLink and Infiniband hardware, combined with software like NCCL, enable both vertical (scale-up) and horizontal (scale-out) scaling. This communication technology is essential to the GB200 NVL72, which integrates 72 GPUs into a single, cohesive unit—a major leap beyond the traditional eight GPU nodes. Furthermore, Nvidia is exploring Ethernet alternatives like Spectrum-X.
Yet AMD is developing competing technologies for both scale-up and scale-out through industry-standard initiatives. UALink aims to provide a high-speed interconnect for chiplets and accelerators within a system (comparable in some ways to NVLink for scale-up), while UltraEthernet targets high-bandwidth networking between systems for scale-out (competing with Infiniband).
Additionally, AMD acquired ZTSystems to help with systems-level design and integration, and that’s already making an impact according to Forrest Norrod (AMD EVP and GM of the Data Center Solutions Business Group). Per FierceElectronics,
“We're still two different companies, so we can't operate as one yet, but we can put in place strong contractual agreements that allow us to engage resources on forward-looking products,” Norrod said. “We have already done so on 355 (MI355X), on the… 400 (MI400X)series, and quite candidly, beyond, so… you will see some contribution from the ZT System resources in the 350 series systems… but certainly see a major contribution from the ZT systems engineering teams on 400 and beyond.”
5) System Level Flexibility
We made the case for chip level flexibility of GPUs earlier: GPUs have a large surface area for absorbing software innovations.
But then we said it’s all about system performance — networking and software included.
What about system-level flexibility?
Could AMD differentiate by creating datacenters that can absorb and support system-level innovations?
From Norrod via the FierceElectronics article,
“We don't want to take the approach that we have a one size fits all. We're not trying to take the Henry Ford approach of ‘You can have your hyperscale data center rack any color you want as long as the color is black.’ We're investing in our systems engineering not only to produce a great set of basic designs and elements, but also to allow others in the ecosystem to do variations, to add their own value. That actually takes a little bit more engineering upfront to add the hooks and design the components so that others can do that, but we think by doing so we better harness the engineering talent across industry to accrue value.”
Yet It’s A Long Grind
If I haven’t repeated it enough, this will all take time.
Software and hiring take time, and clearly AMD is working on it:
Competing on performance at the system levels takes time.
Ramping MI350 and then MI400 takes time.
Networking improvements, especially with industry consortiums, take time.
Fully integrating and leveraging acquisitions like ZTSystems and SiloAI takes time.
The continued shift from training-dominated demand to inference-heavy demand takes time.
Using flagship customers as references to win other customers takes time.
Challenges and Next Steps for AMD
Behind the paywall is my take on Instinct’s opportunity, including a few outstanding challenges and potential next steps for AMD.