Is Intel's CPU Role Diminishing In The Age of AI?
Is Intel's Datacenter AI Tailwind Slowing? What Pat G Says.
In this article, we unpack Vivek Arya’s pointed question from Intel’s Q1 2024 earnings call: Is Intel's CPU undifferentiated in a Gen AI system?
We’ll first explore Intel’s broader server CPU headwinds and tailwinds, then discuss the role of the CPU server in a Gen AI setup, and finally analyze Intel CEO Pat Gelsinger’s response to Vivek’s question.
Here’s the full question to get us going:
Vivek Arya -- Bank of America Merrill Lynch -- Analyst
Thanks for taking my question. Pat, just a conceptual question. In a Gen AI server with accelerators, how important is the role of a specific CPU? Or is it easily interchangeable between yours, or AMD’s, or Arm’s? I guess the question is, if most of the workload is being done on the accelerator, does it matter which CPU I use? Can that move toward the gen AI servers, essentially shrinking the TAM for x86 server CPUs? Because a number of your cloud customers have announced Arm-based server alternatives. So, I'm just curious, how do you think about that conversion over to Gen AI, and what does that mean for x86 server CPU TAM going forward?
Let’s zoom out for newer folks and give some context.
Headwinds
Three broad trends are negatively impacting Intel’s server CPU business today.
1) AMD’s increasing competitiveness
A long-running concern for Intel is AMD’s increasing competitiveness in the x86 server market. Intel still holds a strong majority, but any share loss hurts; volume is crucial in a scale-driven industry like semiconductors, particularly as an integrated device manufacturer (i.e., Intel manufactures its own chips).
Why is AMD slowly taking share? Here’s AMD’s CEO Lisa Su explaining during AMD’s Q1 2024 earnings call:
We believe we gained server CPU revenue share in the seasonally down first quarter. Given our high core count and energy efficiency, we can deliver the same amount of compute with 45% fewer servers compared to the competition [Intel], cutting initial CapEx by up to half and lowering annual OpEx by more than 40%.
If you’re wondering how AMD outperforms Intel so drastically, one major contributing factor is the underlying semiconductor fabrication technology. AMD’s CPUs are fabricated by TSMC, and Intel’s foundry has struggled against TSMC as of late:
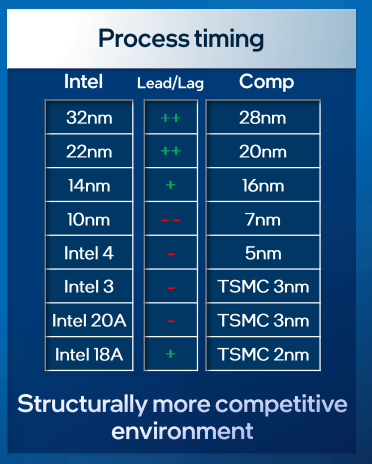
Intel is working to close this gap.
Additionally, AMD’s competitiveness pushed ahead due to an innovative architectural approach: chiplets.
This conversation between Sam Naffziger and Mark Papermaster explains chiplets and why they matter. They also describe the organizational challenges AMD overcame to transition from monolithic to disaggregated designs successfully.
AMD took the gamble first and it paid off, and Intel has followed suit.
2) Arm-based alternatives
Another headwind for Intel’s CPU server business is the rise of hyperscalers making their own chips, shifting spending away from Intel.
The first mover in this space was AWS in 2018 with Graviton. As Peter DeSantis explained in this AWS re:Invent video,
We've been very excited about our ability to roll out hardware innovation faster, but we asked ourselves if could we do more to accelerate. We started working with Annapurna Systems to deliver an early version of our Nitro system several years ago. We were so impressed by the team in the technology that we acquired the Annapurna systems team in early 2015. While we've been really excited about the team's vision and progress on the Nitro system, we asked them to start thinking about what an AWS-designed and built server processor might look like.
Tonight I'm excited to announce the EC2 A1 instance, which is powered by the AWS Graviton Processor. By focusing specifically on scale-out workloads and just the functionality required for the AWS environment, the A1 delivers up to 45% lower cost for some of your workloads. The AWS server processor used to power the A1 instance is the AWS Graviton Processor from Annapurna Labs. The Graviton processor is based on a 64-bit Arm architecture and features 16 cores per processor.
AWS acquired Annapurna Labs, a fabless semiconductor company, and built its own Arm server. The chip has a significant TCO advantage and is used by external customers and AWS’s internal workloads.
Fast-forward six years, and Graviton is still alive and kicking on version 4. Microsoft and Google have followed suit, building their own Arm-based CPU servers (Microsoft Cobalt and Google Axion).
This is a clearly a headwind for Intel’s CPU server sales.
3) Shifting budget from data center CPUs to AI accelerators
The newest headwind for Intel: Nvidia’s AI servers are gobbling up everyone’s budgets, leaving less to spend on traditional CPU servers.
Even if we’re in an AI data center bubble and the buying frenzy levels off, signs point to a “new normal” with an increased share of the enterprise budget spent on parallel computing.
Tailwind
Whew, that’s a lot of headwinds. Now it's time for some good news.
CPUs in AI Data Centers
A silver lining for Intel is that they can participate in the Nvidia GPU server explosion because Nvidia’s parallel computing systems still require CPUs.
From Nvidia
Head nodes serve various functions:
Provisioning: centrally store and deploy OS images of the compute and other various services. This ensures that there is a single authoritative source defining what should be on each node, and a way to re-provision if the node needs to be reimaged.
Workload management: resource management and orchestration services that organize the resources and coordinate the scheduling of user jobs across the cluster.
Metrics: system monitoring and reporting that gather all telemetry from each of the nodes. The data can be explored and analyzed through web services so better insight to the system can be studied and reported.
Two Architectures, One Intel
Broadly speaking, there are two accelerator system architectures in the wild, only one of which uses x86 CPUs:
Traditional head node + PCIe switch connected GPUs ✅
Tightly coupled CPU + GPU ❌
The traditional head node approach looks like this:
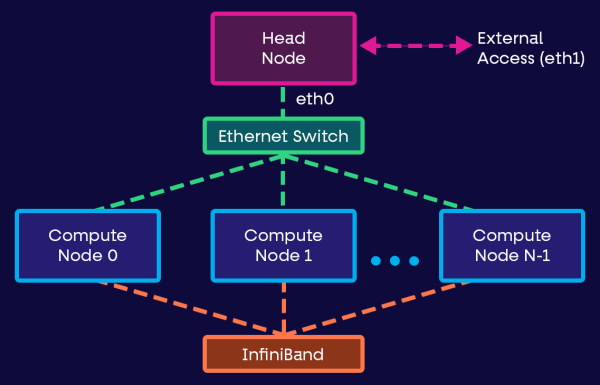
Nvidia’s Grace Hopper “Superchip” takes the tightly coupled approach, reducing latency between the CPU and GPU. HPC Wire explains
Eliminating the PCI Middleman
Before Grace Hopper, CPUs (usually X86) used one or more PCI-bus-based GPUs. These additional GPUs must communicate over the PCI bus and, therefore, create two or more distinct memory domains: the CPU domain and the GPU domain. Data transfer between these domains must travel across the PCI bus, which often becomes a bottleneck.
Grace Hopper has connected the CPU and GPU using the NVLink-C2C interconnect providing a single shared memory domain. That is a memory-coherent, high-bandwidth, and low-latency interconnect. It is the heart of the Grace Hopper processor and delivers up to 900 GB/s total bandwidth.
Note that Nvidia recently launched a successor to Grace Hopper called Grace Blackwell. Grace Blackwell has the same Grace GPU but has two Blackwell GPUs.
Impact to Intel
With the traditional setup, companies often choose an Intel Xeon CPU server for the head node, so Intel still captures some of the accelerated computing spend. Yet Intel only participates in the traditional head node setup, as the tightly coupled approach offered by Grace Hopper and Grace Blackwell uses Arm CPUs.
Pat’s Response
Now that we’ve covered the basics let’s dive into Vivek’s question and Pat’s response.
Here’s Vivek’s question again:
Thanks for taking my question. Pat, just a conceptual question. In a Gen AI server with accelerators, how important is the role of a specific CPU? Or is it easily interchangeable between yours, or AMD’s, or Arm’s? I guess the question is, if most of the workload is being done on the accelerator, does it matter which CPU I use? Can that move toward the gen AI servers, essentially shrinking the TAM for x86 server CPUs? Because a number of your cloud customers have announced Arm-based server alternatives. So, I'm just curious, how do you think about that conversion over to Gen AI, and what does that mean for x86 server CPU TAM going forward?
Vivek asks four interesting questions:
How important is the role of the CPU in the Gen AI server?
Could an Intel head node be swapped out for AMD?
Could an x86 head node be swapped out for Arm?
If the previous is true, how will that impact x86 server TAM?
CPUs: Beyond The Head Node
Pat started with Vivek’s first question but didn’t discuss the head node. Instead, he brought up a GenAI use case called retrieval augmented generation (RAG).
RAG Evangelism
Pat Gelsinger. Yeah. Thanks, Vivek. We spoke at our Vision event about use cases like RAG, retrieval augmented generation, where the LLMs might run on an accelerator, but all of the real-time data, all of the databases, and all of the embeddings are running on the CPU. So, you're seeing all of these data environments, which are already running on Xeon and x86, being augmented with AI capabilities to feed an LLM, and I believe this whole area of RAG becomes one of the primary use cases for enterprise AI.
And if you think about it, an LLM might be trained with one-, two-year-old data, right? But many of the business processes and environments are real-time, right? You're not going to be retraining constantly. And that's where this area of the front-end database becomes very prominent. All of those databases run on x86 today. All of them are being enhanced for use cases like RAG.
And that's why we see this unlock occurring because the data sits on-prem, the data sits in the x86 database environments that are all being enhanced against these use cases.
Pat believes in a future where enterprises create intelligence from their own data using RAG. He points out that RAG will always require feeding data from an existing database, which is most likely x86 and on-prem. On-premises is code for “sticky”; it’s likely not going to the cloud.
LLMs on Xeon?
Pat then added a very interesting interjection:
And as we've shown, we don't need accelerators in some cases. We can run a 70 billion parameter model natively on Xeon with extraordinary TCO value for customers.
Pat’s “oh hey, you can run the LLM piece of RAG on Xeon too” caught me by surprise.
I’m sure it’s possible, but I assume the performance would be painfully slow. Enterprise RAG can be used in ways similar to a search engine, and everyone has very high expectations for search engine response times (thanks Google).
I personally would love to see Intel demo RAG using only Xeon hardware. If it turns out to be performant enough, Intel’s marketing should loudly trumpet, “Enterprises can cost-effectively host RAG using the Xeon hardware they’re already comfortable with.”
Stickiness of Xeon
After this quick aside, Pat got back on track with more supporting arguments for the “stickiness” of x86 servers in the enterprise RAG use case.
And furthermore, all of the IT environments that enterprises run today, they have the security, they have the networking, they have the management technologies in place. They don't need to upgrade or change those from any of those use cases. So, we see a lot of opportunity here to build on the enterprise asset that we have with the Xeon franchise, but we're also going to be aggressively augmenting that.
Pat’s long response to “how important is the role of a specific CPU in Gen AI” can basically be summarized as “we believe server CPUs will continue to contribute to Gen AI workloads, even if not as the head node”
Note that Pat’s “beyond the head node” arguments were generic to x86, leaving the door open for AMD.
Head Node
No Answer Yet
Next, Pat mentioned the head node very briefly, but only to affirm that Intel is often the vendor of choice.
So, we see a lot of opportunity here to build on the enterprise asset that we have with the Xeon franchise, but we're also going to be aggressively augmenting that, and we're commonly the head node, even when it's other accelerators being used or other GPUs being used
Gaudi BTW
Pat then continued with a nod to Gaudi.
and as we've described, Xeon plus Gaudi, we think is going to be a very powerful opportunity for enterprises.
This is Pat saying, “Xeon will be that front-end database for RAG, and don’t forget the LLM can run on Gaudi!” In the modular future that Lisa Su envisions, some enterprise AI purchasing decisions will choose “good enough” performance and optimize for other things like TCO and ease of integration. If Gaudi is “good enough,” Intel should be able to go to their stickiest customers and sell Gaudi based on the interia arguments Pat laid out above. “You’re already comfortable managing Xeon. You’ve got the security figured out. Simply drop Gaudi in there, and you’re off to the races…”
Closing
Finally, Pat made a closing summary, re-emphasizing his belief that enterprise Gen AI will use on-premises data, which plays into Xeon’s hands.
So, in many of those cases, we see this as a market lift, new applications, new use cases, new energy coming to the enterprise AI.
Here we are in year 23 of the cloud. And while 60% of the workload has moved to the cloud, over 80% of the data remains on-prem under the control of the enterprise, much of that underutilized in businesses today. That's what Gen AI is going to unlock. And a lot of that is going to happen through the x86 CPU and we see a powerful cycle emerging. And I would just point you back to what we described that vision. This was a great event, and many customers are seeing that value today.
Non-Answer
Vivek asked a rather pointed question: why should the head node be Intel?
Pat did not give a good argument for why the head node must be Intel.
Maybe there aren’t any good reasons?
Substitutable Head Node
Since Pat didn’t address Vivek’s questions, I’ll take a stab!
1) In a Gen AI server with accelerators, how important is the role of a specific CPU?
The “jobs to be done” by the CPU are important, but the particular CPU itself isn’t important. The workloads could be carried out on a cheaper CPU; they don’t need to be done on a high-end x86.
In fact, the hardware doesn’t need to be a CPU at all; a custom ASIC could do the job if it made economic sense.
2) Could an Intel head node be easily interchangeable with an AMD server?
Definitely. The software should transition seamlessly since both are x86. There are essentially no switching costs.
3) What about swapping that x86 server for an Arm server
It’s possible. This approach requires more effort to ensure the software stack runs smoothly on an Arm server, and many enterprises do not have that skillset readily available. The switching costs would be higher for those enterprises.
4) If Google, AWS, Microsoft are making their own Arm CPU servers that are interchangeable as the head node, how will that impact x86 server CPU TAM?
These companies have very large AI accelerator clusters and could save the most from swapping out Intel head nodes for their own Arm-based servers. Moreover, they have the software skills to port or smooth out issues with Arm, as they are already doing this when they move software workloads to their Arm-based servers.
How will that impact x86 server CPU TAM? It’s fair to wonder if the future AI accelerator data centers with the largest hyperscalers will either
Have a traditional head node set up with their own Arm servers
Use the tightly-coupled Grace Blackwell approach (also Arm)
In such a future, the TAM of the x86 CPU server will clearly decrease. Of course, the interesting question is: how much? I haven’t estimated the current size of the CPU head node market, but it should be doable. I’ll leave that exercise to the reader 😊
It’s unlikely Intel would claw that back by trying to convince these customers to use Xeon + Gaudi, as these customers are optimizing for the highest performance, which will likely always be Nvidia.
Tailwinds are changing directions
Arm CEO Rene Haas agrees. During their May 2024 earnings call, he added to the conversation, explaining the power of the tightly-coupled approach, why he believes customers are moving in this direction, and the positive impact for Arm:
Secondly, and I think we chatted about this during the IPO process with Grace Hopper, but I think now with NVIDIA's most recent announcement, Grace Blackwell, you are going to see an acceleration of Arm in the data center in these AI applications.
One of the benefits that you get in terms of designing a chip such as Grace Blackwell is, by integrating the Arm CPU with the NVIDIA GPU, you are able to get an interconnect between the CPU and the GPU that allows for much higher access to memory, which is one of the limiting factors is for training and inference applications. In a conventional system where you might connect to an x86 externally, you have to do that over a PCIe bus, which is much slower. So, by using a custom bus in the NVIDIA example, like NVLink, you get much higher memory bandwidth.
I think what that is going to mean is that Arm adoption in the data center will probably increase faster than the numbers that we had indicated, but we're not saying anything official right now.
It seems Rene believes the Gen AI CPU tailwinds are changing directions, away from x86 and toward Arm.
Final Thoughts
When I listened to this call, I was honestly annoyed that Pat gave Vivek a non-answer. But after writing this post, I’ve come to appreciate Pat’s real-time ability to recognize the question as a “trap” and navigate around it.
It’s a “trap” in the sense that Intel isn’t differentiated in the head node, but do you want to admit that on an earnings call? Instead, Pat took the wheel and steered in a different direction, painting a picture of where he believes Intel CPUs will play in Gen AI going forward.
To continue this deep-dive, see the next post:
Intel CPU follow-up, Nvidia Superchips, AMD MI300A
If you enjoyed this post, consider subscribing to receive some or all upcoming posts!