Rethinking AMD
Modular vs integrated, "right tool for the job" value prop, rethinking the org chart
Let’s conduct a thought experiment about AMD and see what insights arise.
We’ll imagine a generative AI future, ask if AMD is positioned to succeed, explore what steps they could take to improve their odds, and discuss challenges they would face.
Along the way, we’ll discuss modular vs integrated AI semiconductor companies.
An AI Future
Imagine the not-too-distant future where generative AI is woven into the fabric of daily life. Like mobile phones, gen AI transformed from a peripheral tool to an essential companion.
Hands-free assistance works: “Hey Glasses, decline this call from my mom, but snap a photo and tell her I’m in the middle of teaching her granddaughter how to ride a bike!”
Gone is the chaos of incessant push notifications, arrays of phone apps to hunt and peck through, and overflowing email inboxes. Smartphone UIs are now a minimalist interface powered by a friendly AI assistant that calmly surfaces what matters when it matters.
Office life is equally transformed. Employees leverage AI agents to offload busy work so they can spend more time innovating, deeply thinking, and serendipitously interacting.
Individuals and small teams can do more than ever before, driving a boom in the solopreneur and small business economy.
At the heart of this future, from home to office to cloud, is AMD.
How Might This Future Come True for AMD?
Betting on Gen AI as a transformative foundational technology, not just a passing fad
Let’s think through how AMD can be a central player in this future.
First, it feels obvious to state but is worth explicitly mentioning: Executive leadership must have a strong conviction that this generative future will happen.
Belief is the first step, the second is investment.
If we’re entering a strategic inflection point, then AMD must wager its entire focus on generative AI — from product roadmaps to R&D to sales and marketing.
A strategic inflection point is a time in the life of business when its fundamentals are about to change. That change can mean an opportunity to rise to new heights. But it may just as likely signal the beginning of the end. -Andy Grove
In light of the conclusion above, let’s take a look at AMD to see if they are fully committed to a Gen AI future. If not, we’ll explore steps they could take.
AMD’s Present Strategy
AMD’s current approach integrates AI as an afterthought within traditional segments.
AMD has built compelling AI products within some of its business units, demonstrating some level of belief in generative AI as a strategic inflection point. At the same time, this could also be interpreted as putting an AI spin on existing products.
The vibe I get from AMD’s current approach is “one foot in the future, one foot in the past”, which comes across in their public communications too.
Take the 2023 annual report as an example, where the following slides illustrate:
We have a broad portfolio
Here are our business units and their products
We have some AI within those business units!
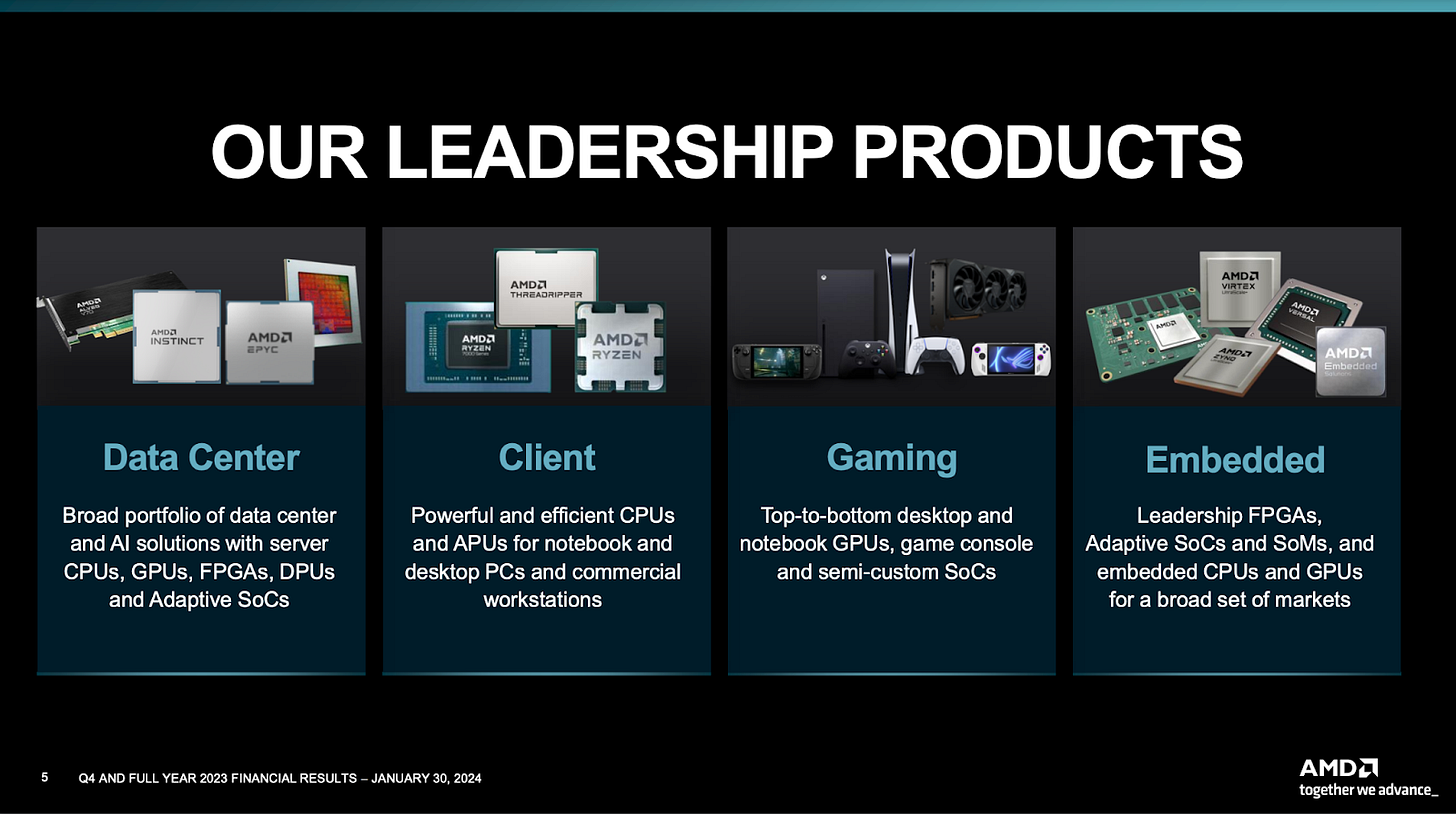
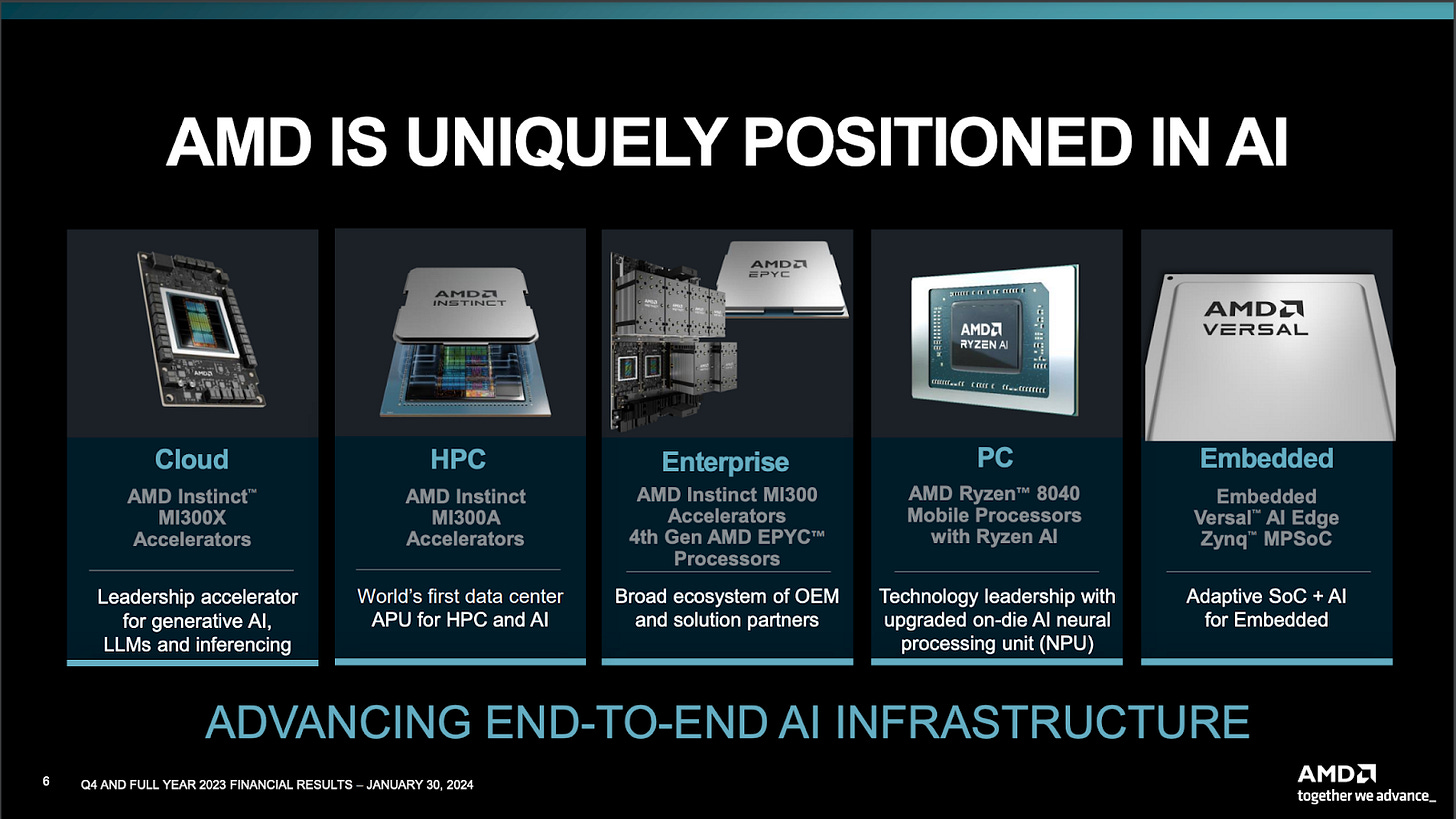
AMD’s current approach lacks the clarity and strategic focus necessary to inspire employees, customers, and investors that the future is generative and AMD is ready for it.
If AMD believes in a generative future, it needs a compelling vision with a business structure designed to execute it.
What is the appropriate business structure then?
Business Model
A prerequisite for rethinking AMD’s business structure is an articulation of AMD’s business model in this generative future.
Let’s look at modular and integrated approaches for AI companies.
My old boss, Jim Barksdale, used to say there’s only two ways to make money in business: One is to bundle; the other is unbundle. -Marc Andreesen
Integrated
Integration occurs when a single company owns multiple components of the AI value chain.
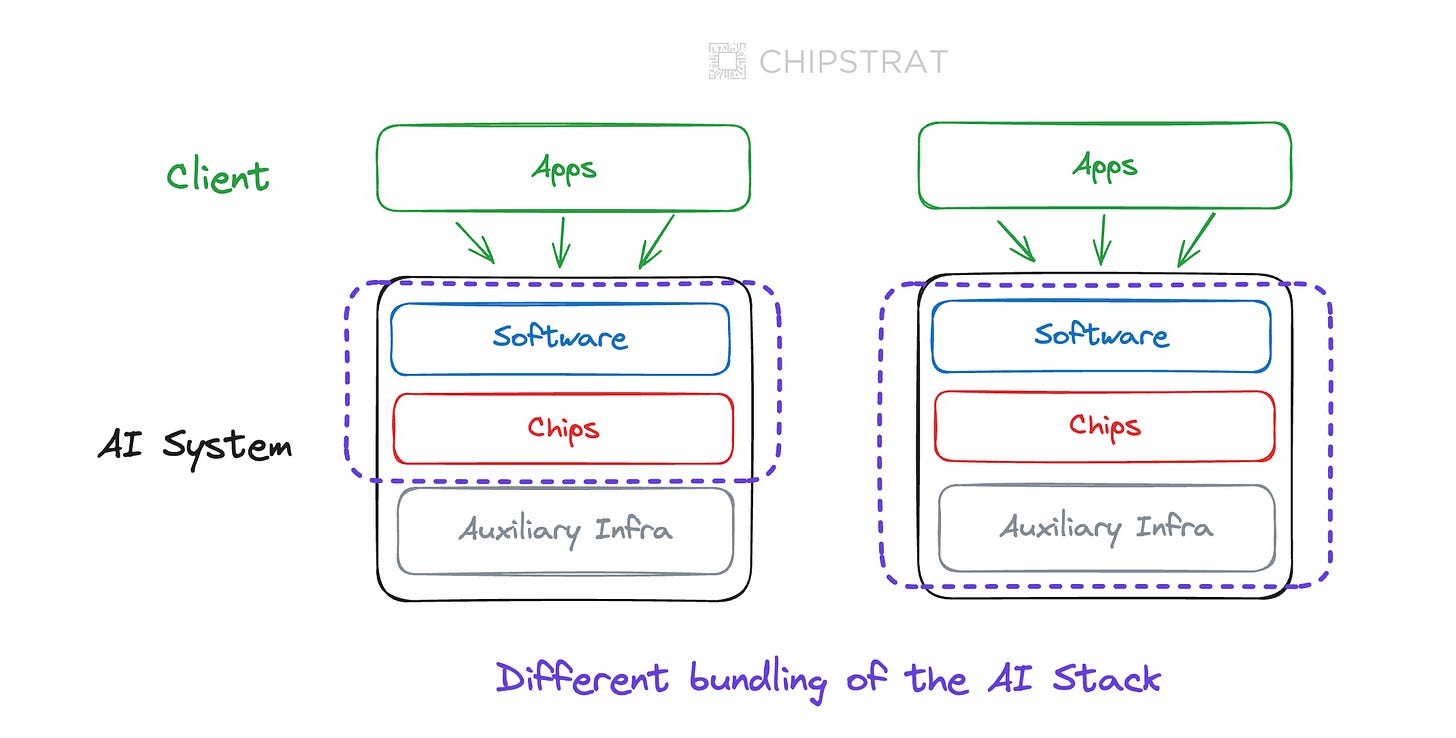
The obvious example of a vertically integrated semiconductor company is Nvidia.
Take Nvidia’s cloud offering as an example of integration.
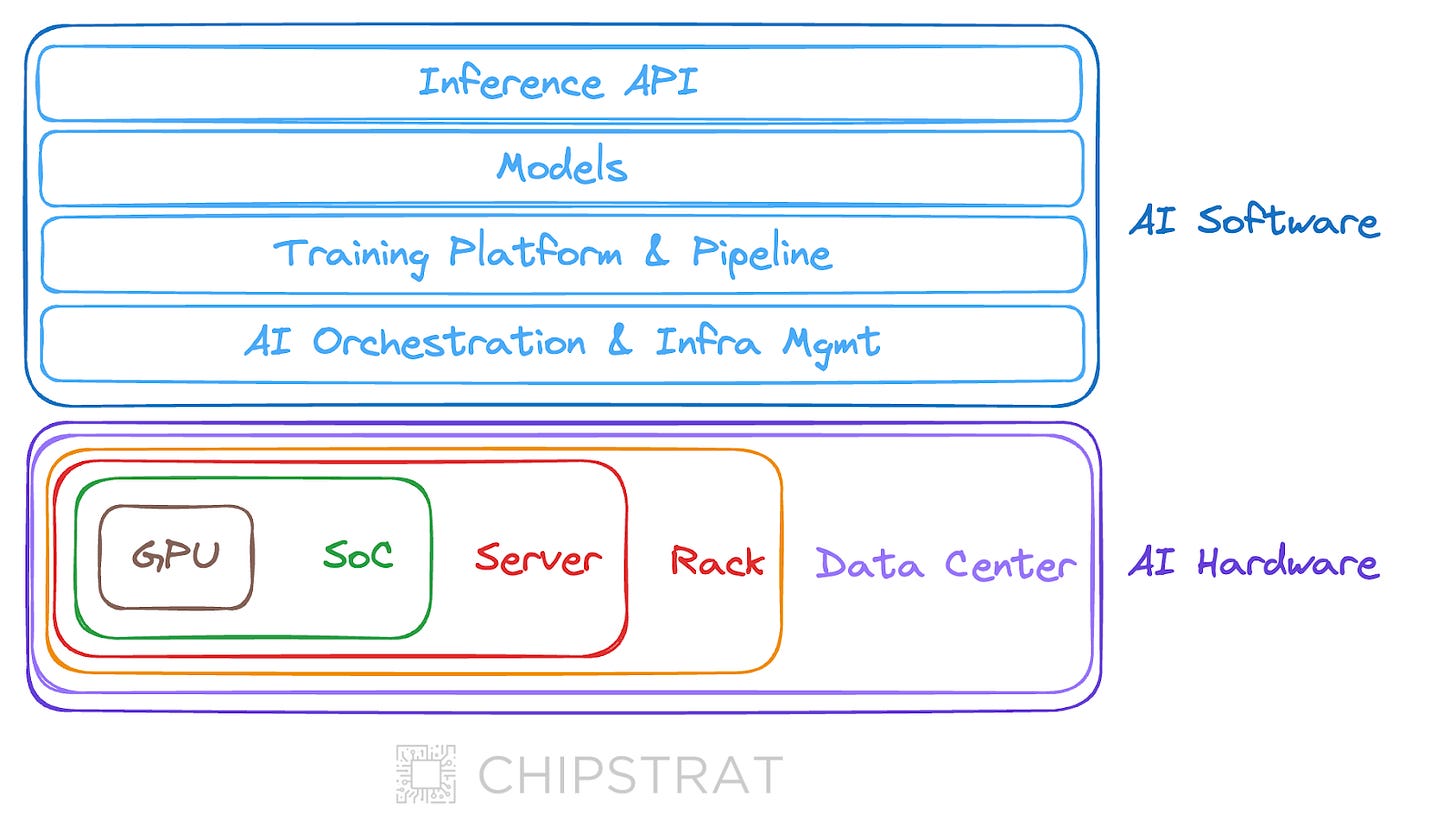
Nvidia has integrated up the hardware stack, from GPUs up to complete data centers, including designing auxiliary components like the SoC CPU (Grace) and networking technologies (NVLink, NVSwitch, Infiniband). They source other components to assemble each subsystem, all the way up to the data center — Nvidia DGX SuperPod, “The World’s First Turnkey AI Data Center”.
Nvidia’s integration extends beyond hardware, with a complete AI software layer on top to enable ML developers to train, fine-tune, and deploy AI models. Finally, they explicitly call out their implementation service & support as an integrated “feature” of the enterprise system.
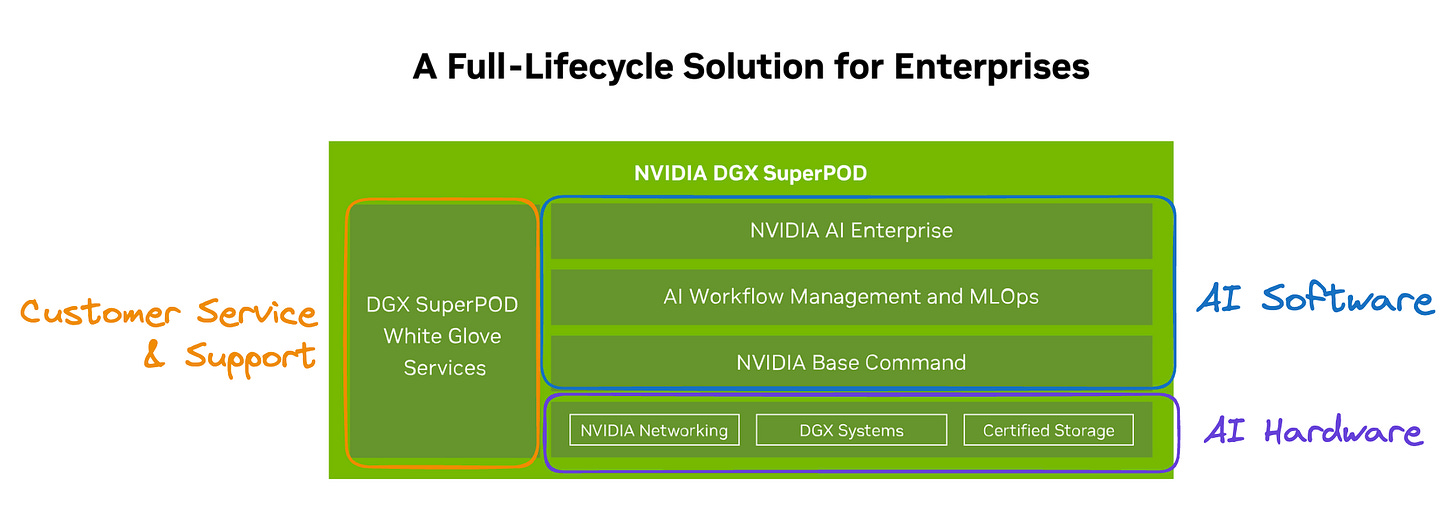
Vertical integration delivers advantages for both customers and the company. Customers enjoy enhanced user experiences, as software “just works”, minimizing time-to-deployment. Meanwhile, the company benefits from increased value capture, higher margins, and robust customer retention due to greater switching costs.
Common analogies of this hardware and software vertical integration approach include IBM’s mainframes, Apple’s consumer products, and Tesla EVs.
Take note that companies succeed when they choose either a vertical or integrated approach, but typically not both – either modularize and compete on cost in the low end of the market or integrate and sell a premium offering to the high end. Yet at this moment, Nvidia is taking both a horizontal and vertical approach, allowing customers to buy subsystems or the whole enchilada. A deeper dive is warranted, but Nvidia can pull off both right now mainly due to a lack of substitutes.
Modular
The modular alternative is to focus an AI business on certain components or subsystems within the AI stack.
TechFund has a great explanation of modular AI from an article contrasting Broadcom and Nvidia
The opposing view for the future of the AI datacenter was provided by Broadcom. Rather than having everything vertically integrated, Broadcom is seeing a plug and play world, where components of different manufacturers can be swapped in and out of the AI datacenter at will from GPUs to CPUs, NICs and switches, communicating over open standard ethernet…. every player in the value chain is focused on their task.
The customer benefits of modularity include flexibility and choice but at the cost of user experience and often performance. A plug-and-play situation creates a proliferation of system configurations, increasing the chances that the software doesn’t “just work”. Off-the-shelf components may not be fully optimized to meet the performance of a fully integrated design.
Why Modular Will Exist
It’s reasonable to conclude that any company that wants to compete in AI must build vertically integrated data center solutions, especially because the market leader Nvidia is vertically integrated.
“Forget GPUs, you’re competing at the rack. Plus Nvidia has NVLink, Infiniband, and CUDA”.
Of course, this implicitly assumes that edge inference will be negligible. There are valid technical feasibility reasons why people draw that conclusion, but as a counterargument, there are customer and business needs that will demand edge inference. Let’s hold that conversation for another time.
Competitors indeed need to ensure their hardware is competitive in rack and data center solutions, and networking is a crucial piece of that. It’s also true that competitors must ensure AI software runs seamlessly on their hardware.
But modular companies can meet these needs too.
Let’s dig into customer demand for a modular approach.
GPUs Won’t Always Be King
I know I’m a broken record here, but the future will move beyond GPUs.
GPUs are like mountain bikes. Mountain bikes can take you to your destination faster than walking, and they are versatile enough to work anywhere. If everyone was walking, and someone started selling mountain bikes, you better believe there’d be lines out the door of people trying to buy mountain bikes.
Today, Nvidia’s vertically integrated, GPU-centric cloud offerings underlie the majority of generative AI training and inference. Nvidia sells mountain bikes, and they’d like you to believe mountain bikes are the end-all-be-all.
But what if you want to compete in a road race? Mountain bikes aren’t the best choice for that. They've been designed for versatility, requiring a heavier frame and big tires. On the other hand, a road bike’s lighter frames and narrow tires translate to fast and energy-efficient road racing.
The point of this analogy: customers will demand the right tool for the job. This will create a broader solution space than just throwing premium GPUs at everything.
Competitiveness in AI hardware won’t always mean raw FLOPS performance, but instead customers will make decisions weighing other metrics like performance per Watt (PPW), total cost of ownership (TCO), software compatibility, support, and ease of deployment.
Integration Point Drives Modularization
A clean integration point will also help drive modularization, or “unbundling”.
For cloud inference, the model is hosted in the data center and served to client apps via an API layer.
This API layer becomes the point of integration.
The hardware underneath is abstracted from the developer, allowing for modularization of the AI stack.
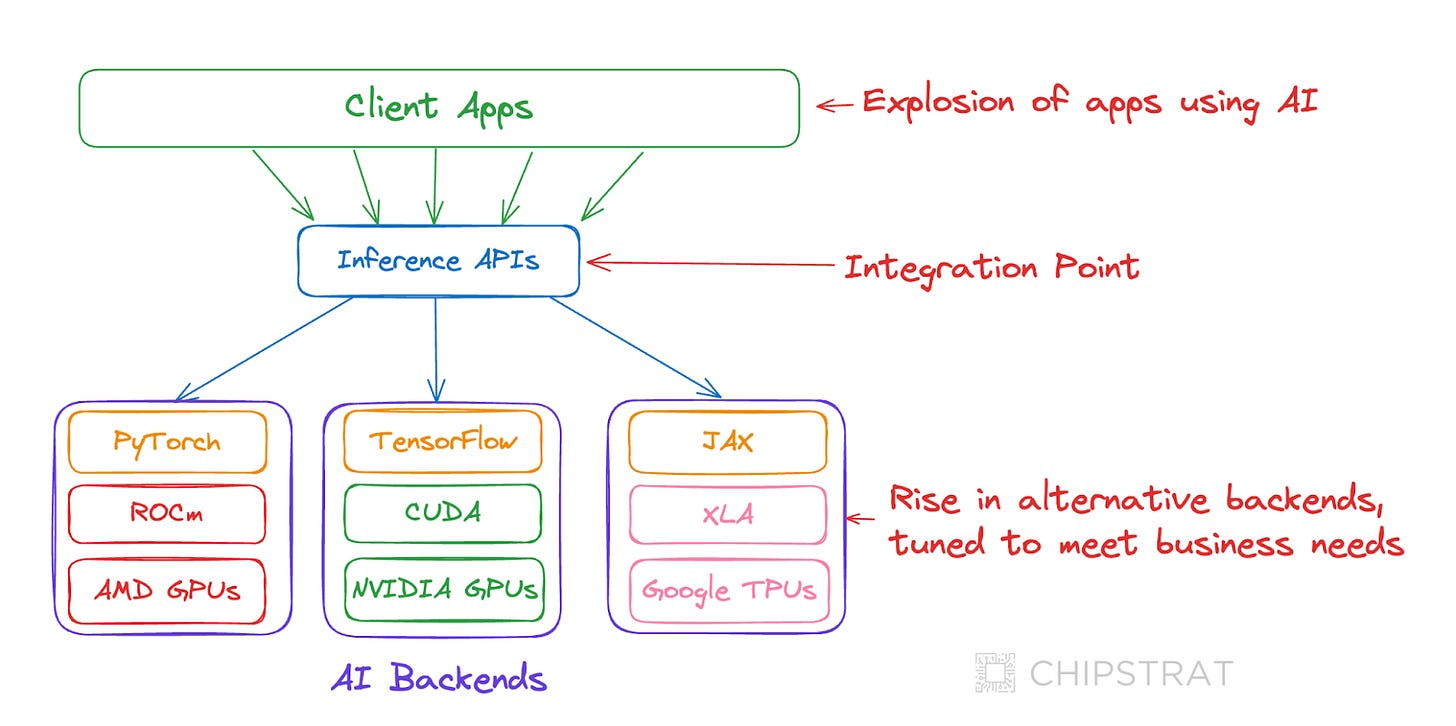
This dynamic is already in motion even though we’re only in the early stages of generative AI adoption. How much more so in the coming years!
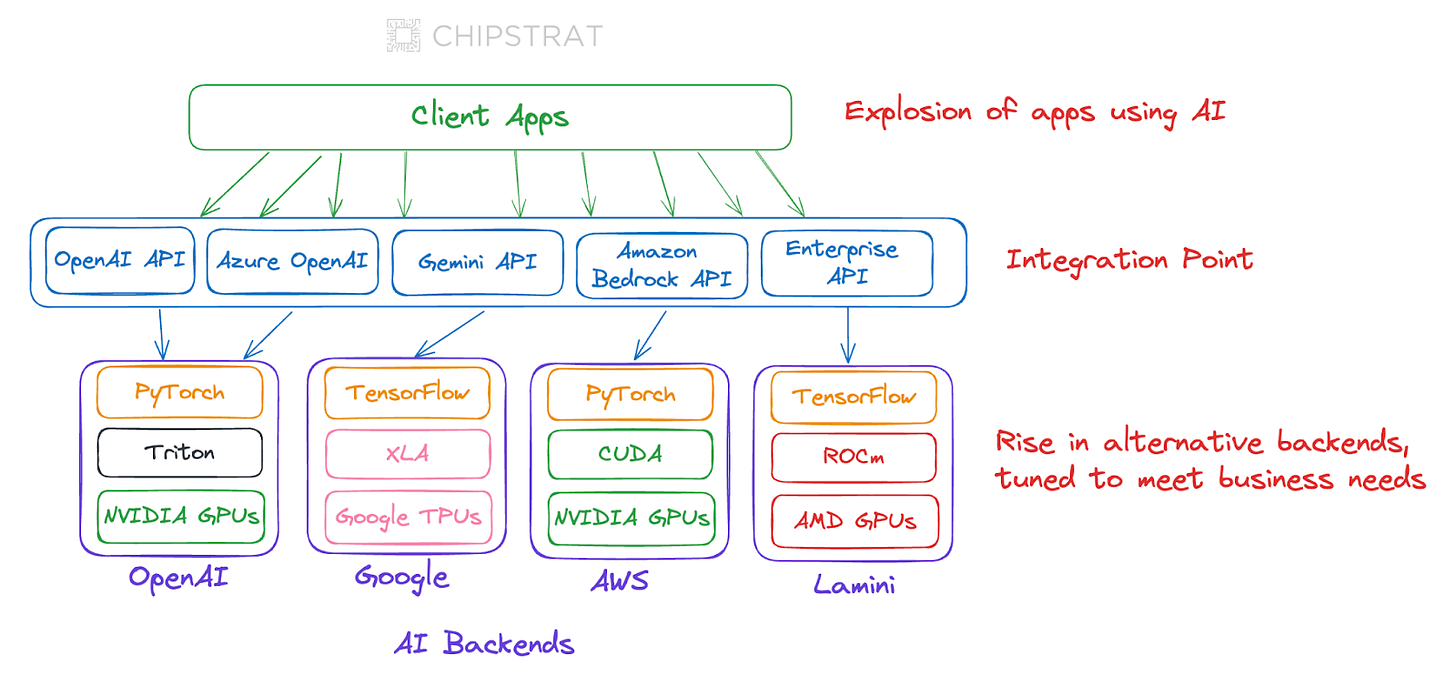
Customer Demands Will Change
Not only will “unbundling” be possible, but customers will demand it.
As generative AI gains widespread adoption, customers will demand more than just raw power. The days of throwing expensive GPUs at every problem are numbered. Skyrocketing costs (CapEx, OpEx) and data center power issues will drive the need for efficiency in AI hardware choices.
Furthermore, while cloud providers make it easy for startups to select top-of-the-line hardware, this is often funded by venture capital. Every growing company has the inevitable "cloud cost reckoning" which will force the company to optimize, shifting their focus from pure performance (FLOPs) to PPW and TCO.
The core business opportunity: Customers will want the "right tool for the job".
Solutions that deliver optimized hardware configurations and cost-effective deployment will thrive.
AMD Should Stick With Modular
Now that we’ve explored AI semiconductor business models, let’s get back to AMD.
A modular approach is the right fit for AMD given their diverse product portfolio and design expertise. AMD can position itself as the ultimate solution provider: come to us with your workloads, and we’ll get you the right-sized systems for your unique requirements.
Thus, in a generative world, AMD's horizontal business strategy should focus on two primary markets: cloud and edge, built upon an open software ecosystem and differentiated by their technology and design prowess.
With this change, AMD’s marketing, sales, and recruiting efforts should explicitly make clear AMD is pivoting from “a hardware business, enabling AI” to “an AI business, with enabling hardware and software”.
It might come off as too simple at first blush, but hang with me. Let’s add detail to the cloud and edge business units.
Modular Cloud Portfolio
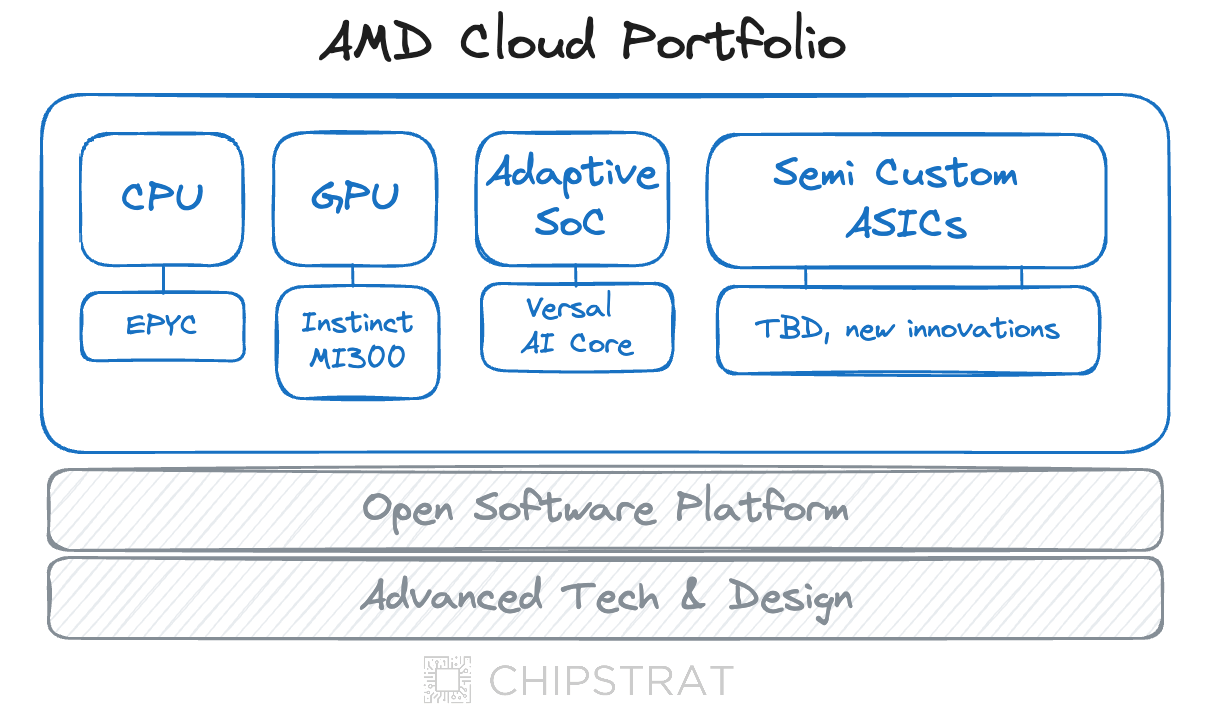
AMD’s cloud business has a variety of products to meet customers' varying needs.
Businesses looking to streamline costs and improve hardware efficiency might consider using AMD's MI300 GPUs and Epyc CPUs in tandem by appropriately offloading lighter inference to CPUs. These CPUs can also run traditional workloads like API services or batch processing work when not being utilized for AI — boosting CPU utilization, reducing GPU needs, and ultimately saving money.
Clouds and hyperscalers who want to reduce power consumption can orchestrate their workloads across AI accelerators and GPUs – for example using GPUs for training, and low-power AI accelerators for inference at scale.
Some customers may choose Adaptive SoCs in the datacenter so they can get ASIC-like speed and power consumption, yet have the flexibility to reconfigure the hardware as Gen AI architectures evolve.
Finally, AMD can build semi-custom datacenter AI accelerator chips to a customer’s specific workload to optimize performance efficiency, akin to Meta and their MTIA2. For example, maybe large tech companies like Netflix would benefit from tailored accelerators for scaled inference but don’t have the desire to build silicon teams like AWS, Meta, and Google.
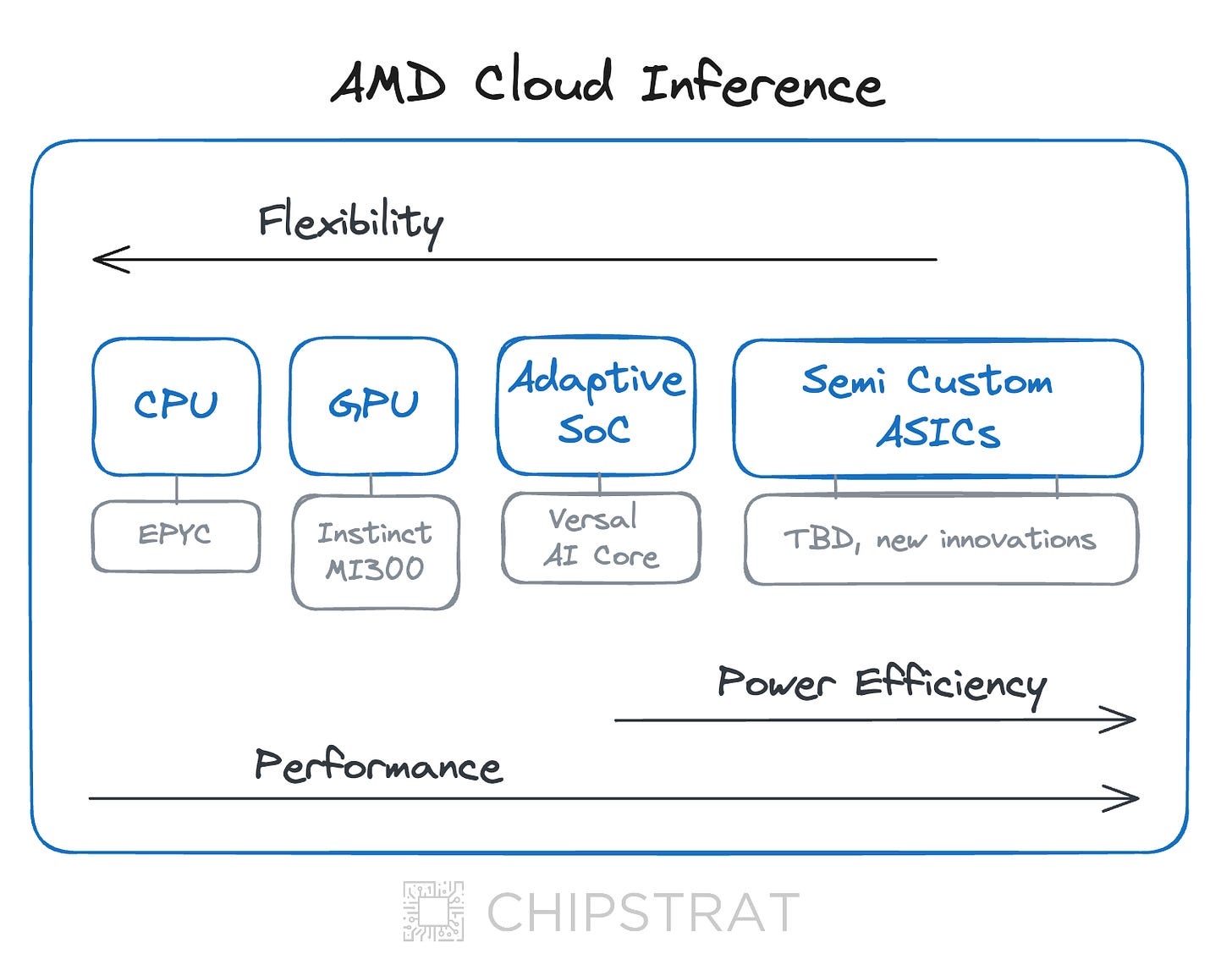
This modular cloud portfolio requires a supportive software ecosystem. By ensuring robust support for core frameworks like PyTorch, TensorFlow, ONNX, and JAX on ROCm, and providing tools like Vitis AI for adaptive SoCs, AMD can create the developer experience necessary to both compete and enable workload distribution across different underlying AMD hardware.
One Stop Shop
AMD's dream as a modular player is to become a one-stop-shop for customers, for example:
This one-stop-shop approach simplifies the process for customers, delivering ease of procurement, technical support, relationship building, a unified software platform, and potential volume discounts or other incentives. Note that these benefits are similar to a subset of those offered by Nvidia’s integrated offering.
Direct sales to on-premises customers maximize these benefits. While cloud deployments may lessen some advantages, customers still experience the core benefits of AMD's low TCO, exceptional PPW, and integrated software platform.
How does legacy hardware fit into the new org structure?
CPUs
The previous hypothetical customer example raises the question, “What about AMD’s CPUs that aren’t running AI workloads? How do those fit into an ‘AI-first’ business unit?”
They fit great!
Datacenter CPUs roll up under the cloud unit while laptops, desktops, and gaming belong to edge.
The cloud and edge businesses don’t imply that “AMD doesn’t support traditional workloads”, but simply exist to reflect AMD’s new strategic focus and drive R&D, marketing, and sales to prioritize AI solutions. Legacy products like CPUs without NPUs will continue to generate revenue, but the goal is to shift investment toward building the future of generative AI and allocate “just enough” investment to legacy products.
Edge
AMD has a broad portfolio of edge solutions as well.
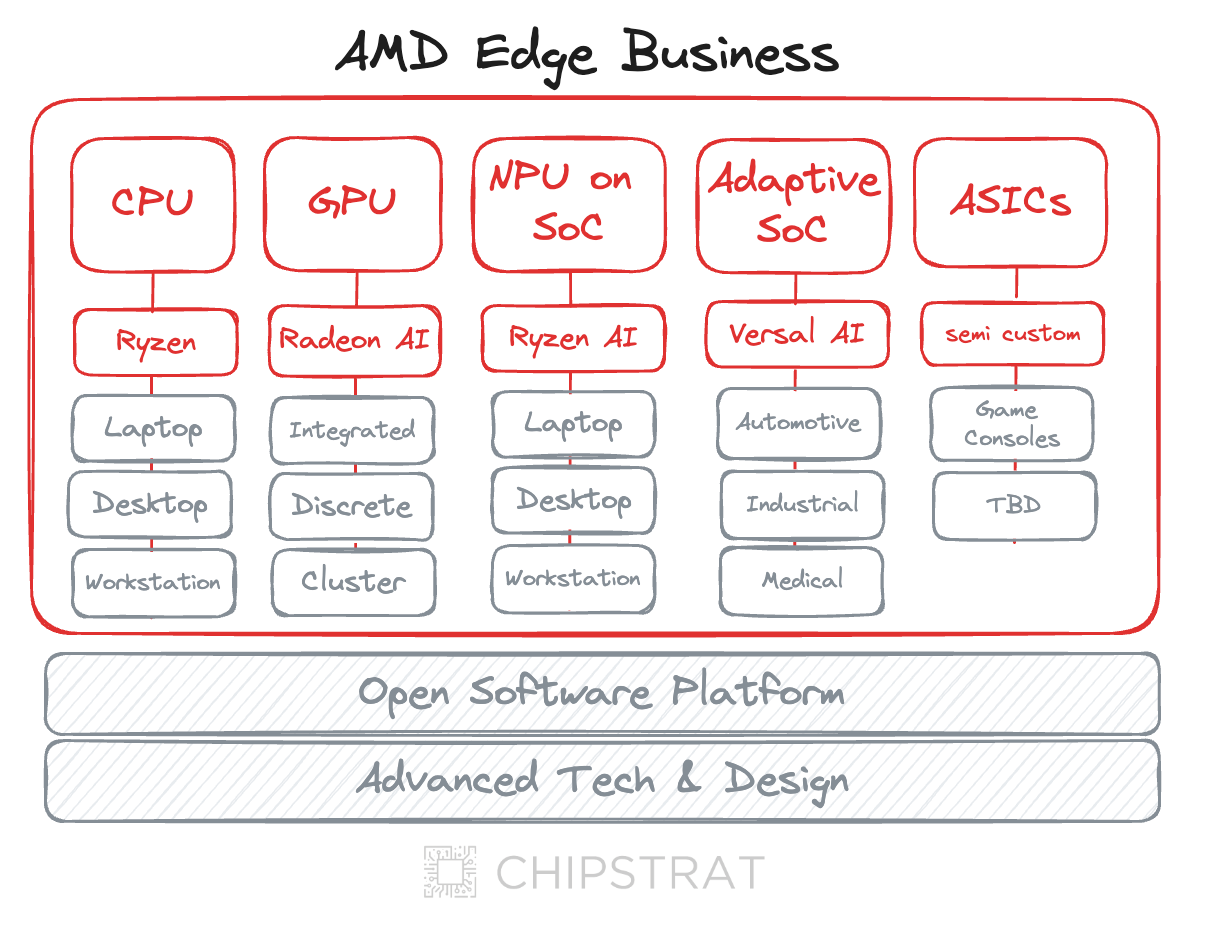
For automotive companies building inference-powered ADAS, autonomous driving, and infotainment solutions, AMD's Adaptive SoCs deliver PPW with the flexibility for over-the-air updates and security patches.
Machine learning engineers have the tools they need for local model fine-tuning and inference using well-equipped workstations with discrete GPUs or on-premise GPU clusters for the most demanding R&D tasks.
For ultra-low latency and power-efficient edge AI – think robot assistants powered by cutting-edge LLMs – AMD could be the solution. A strategic focus on AI innovation opens the door to designing semi-custom chips tailored for these use cases.
What About Gaming?
AMD's strategic shift empowers them to reimagine gaming with AI. By prioritizing AI accelerators on GPUs alongside maintaining their established gaming products, they'll explore how AI can revolutionize the gaming experience.
What About Semi-Custom?
Game consoles are slotted in the semi-custom division of the Edge department. This division is ripe for innovation opportunities that span edge semi-custom ASICs and adaptable SoCs including the aforementioned robotics, but also IoT and wearables.
AMD Must Clearly Articulate Their Differentiation
Strong messaging about the benefits of a new approach
AMD must work hard to clearly articulate they are a modular AI company with a broad portfolio of enabling hardware and software.
Those slides we discussed earlier? With this new focus, AMD can instead tell a convincing story:
We are an AI company
With a broad portfolio of products across cloud and edge
That have fantastic PPW and TCO to meet your unique requirements
Enabled by our broad design IP portfolio, advanced tech, and open software ecosystem
AMD must shed the image of playing catch-up.
They should strive to be recognized as the ideal partner for companies prioritizing solutions that balance performance, cost, and efficiency.
AMD can set itself apart from large competitors like Intel with a focus on innovative AI chip design. Their success with chiplet-based architectures enables flexible, modular designs, accelerating innovation cycles and optimizing efficiency and cost.
As a fabless company, AMD's sole focus on creating world-class AI hardware designs frees them from the complexities of running their own foundry. No need to divert increasingly large amounts of capital and company attention into semiconductor manufacturing; AMD can focus on design, product, and portfolio innovation.
AMD can stand out from AI hardware startups with its established strengths and commitment to supporting customers. AMD’s proven track record of delivering products minimizes the risks associated with less established companies. Strong foundry relationships and a published roadmap backed by ample capital provide confidence that AMD's vision will be realized. Their strategic focus and access to capital also gives AMD a product cadence advantage over startups who can’t afford foundry runs every year. Finally, unlike startups with limited resources and narrow focus, AMD can invest in a broader open software ecosystem across their compute stack.
Lastly, AMD is very vocal about their support of the connective tissue that helps their modular components piece together into a competitive system. They talk about partnering with industry leaders like SuperMicro, Dell, and HPE to provide reference designs. They talk about networking partnerships and investing in open protocols like Ultra Ethernet Consortium. They talk about investments and contributions to software ecosystems like PyTorch, Google XLA, OpenAI Triton, vLLM, and JAX.
Questions, Challenges, Implications
So far, AMD’s reframing as an AI semiconductor company with a broad portfolio across cloud and edge looks quite compelling.
Let’s poke holes in it.
Behind the paywall, we’ll ask questions like:
What about Nvidia?
What’s missing from AMD’s portfolio?
What challenges can we foresee in this future?
What about ARM and RISC-V?
and more!